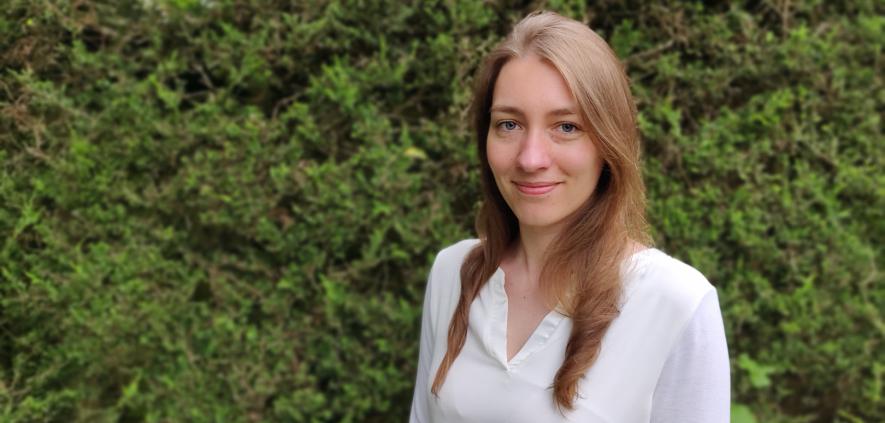
Submitted by Jane Durkin on Wed, 15/09/2021 - 10:52
Artificial Intelligence (AI) is an increasingly central aspect of language science research encompassing many areas from digital humanities and corpus linguistics, NLP applications like speech recognition and chat bots, to the use of machine learning to model human cognition.
Cambridge University is a world-leading centre for language and AI research. In this series of interviews, we talk to researchers from across Cambridge about their work in this field.
Linda Gerlach is a PhD student in forensic phonetics at the University of Cambridge Phonetics Laboratory. Her supervisor is Kirsty McDougall.
Her research focuses on speaker characteristics and forensic phonetics, using traditional phonetic and automatic machine-based techniques.
Linda also works as Research Scientist and Quality Assurance Manager for Oxford Wave Research, a leading audio-processing and voice biometrics company in forensic speech and audio.
Prior to her PhD she completed a master’s in Speech Science at Philipps University Marburg, Germany. For her master’s thesis, “A study on voice similarity ratings: humans versus machines”, she worked in collaboration with the University of Cambridge during an internship at Oxford Wave Research.
Her studentship is based at Selwyn College and is jointly funded by Oxford Wave Research Ltd (OWR) and the Cambridge Trust.
Tell me about your research
My PhD is on speaker characteristics and forensic phonetics.
I’m exploring the relationship between traditional phonetic approaches and automatic machine-based techniques.
More specifically, I'm looking at how to select similar speakers for various forensic purposes, taking into account demographic factors such as age and native language, as well as perceptually salient phonetic and acoustic features.
I'm currently looking at whether voice similarity ratings by human listeners are comparable to similarity estimates from an automatic speaker recognition system.
What inspired this project?
I was already looking at a comparison of listener ratings versus automatic scores in my master's. This was inspired by previous work on automatically selecting similar-sounding speakers that I got involved in during my internship at OWR.
We found a broadly linear significant relationship between listener ratings and the scores we got from the automatic approach.
We published a paper on that last year: Exploring the relationship between voice similarity estimates by listeners and by an automatic speaker recognition system incorporating phonetic features.
My PhD is based on that, looking at how voice similarity operates and defining whether it is possible to find degrees of voice similarity, for example to determine voices that are so similar that they can barely be distinguished.
SEE ALSO: Can voice similarity be assessed using an automatic speaker recognition system?
What is the potential impact of this kind of research?
This is important for forensic phonetic casework including voice parades, forensic speaker recognition and also for voice-related medical applications.
In a case when someone has heard a perpetrator but not seen them and may be able to recognize them from their voice, a voice parade may be carried out.
In a visual parade you have a face or photos of the suspect. Then you have foils, other faces who look similar to the suspect.
For voices there's a similar setup where you have a suspect voice and you need foil voices to sit beside it.
It's difficult to find suitable voices for a voice parade because it's not clear what we as humans perceive as similar and what would be too dissimilar. The phonetic foundations of what makes voices similar is not yet well understood.
A voice parade is also quite rare because it’s so difficult to set up. Currently it's a manual procedure.
At least if we could automate one step in the selection process, you could compare a larger number of speakers in an automatic speaker recognition system and make a preselection of the similar-sounding speakers. There would still be some manual efforts involved, for example to assess whether the speakers’ accents are appropriate for the task, but it would certainly speed up the process.
Assessing the perceived similarity of voices is relevant for other applications as well. This includes forensic voice comparisons, where a speech sample of a suspect is compared to that of a perpetrator and the probabilities of two competing hypotheses are assessed: 1) the two samples come from the same speaker (assessment of similarity) and 2) the two samples come from different speakers (assessment of typicality).
For example if you have a comparison of two male voices and they are both quite a high pitch, do you select a relevant population in which all speakers have a high pitch or not?
Finally, assessing the similarity of voices is relevant with regard to synthesised or cloned voices. For example, when someone ‘loses’ their voice due to an operation or degenerative disease, they may need to rely on synthesised speech that is either trained using old recordings of their voice or – if these are insufficient – recordings of another speaker who sounds similar. To evaluate successful voice synthesis or cloning, a better scientific understanding of voice similarity is needed.
What methods are you using?
For my first study, I drew data from the Improving Voice Identification Procedures (IVIP) and VoiceSim projects, in which the listener experiments had already been run.
There were several speaker groups available, all of which were compared by listeners. I took the same speakers and ran their recordings in the automatic speaker recognition system as a comparison.
There are different feature extraction algorithms and speaker modelling approaches available. I'm currently looking into which are most suitable to choose.
There are two approaches available in the feature extraction part of the automatic speaker recognition system, one is using Mel-frequency cepstral coefficients (MFCCs), so short-term spectral information of the voice signal.
The other is using automatically measured phonetic features – features that have been found to be correlated with perceived voice similarity, for example fundamental frequency (F0), semitones of F0, and their derivatives, as well as formants (F1 to F4).
The spectral ones are more common in automatic speaker recognition and have low error rates there, whereas features such as F0 and formants are traditionally used in phonetic analyses.
I'm planning to look at other clustering methods to get more insight into how voice similarity is structured and what contributes to it.
What does the future hold?
Automatic speaker recognition is now widely used in jurisdictions across the world and currently sits alongside aural-perceptual and measured comparisons by human experts.
My supervisors and I believe objective measures of human-perceived speaker similarity will play an important role in bringing these two approaches together, and increase the adoption of automatic techniques in forensic casework worldwide.
Automatic speaker recognition systems are playing a bigger and growing role in research and linguistics.
There are many things that have not yet been explored using automatic speaker recognition systems, especially with the new algorithms that are being used with deep neural networks.
What opportunities are there in your field for more interdisciplinary work?
I think collaborating with companies or other labs in that field could help us understand what is important for speaker recognition and speaker profiling, or what makes the speakers similar.
As well as work in linguistics and speech science, collaborating with researchers and practitioners in psychology, criminology, and law is crucial for developments in forensic speech science and its application to the legal system. I also see potential in collaborating with researchers working on voice cloning and synthesis.
It would help us also in the forensic part to explain what is going on to people that do not necessarily have the background to understand what is going on themselves.
For example, lawyers or judges will need to understand the evidence, once automatic evidence is allowed to be used.
READ MORE: New PhD studentship in forensic phonetics