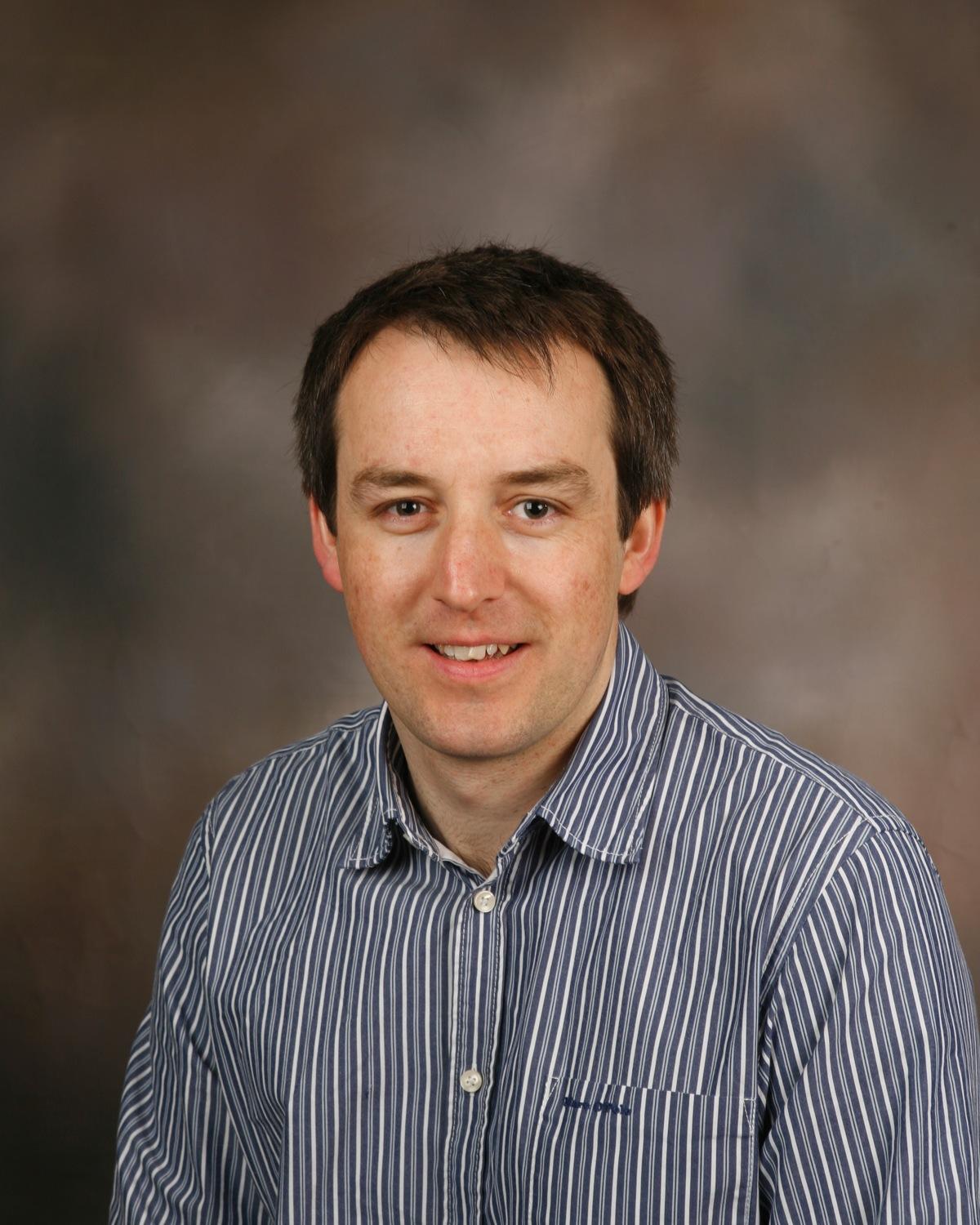
Submitted by Administrator on Thu, 03/05/2012 - 15:03
An interdisciplinary research collaboration on computational semantics by the computer science departments of Cambridge, Oxford, Sussex, York and Edinburgh has just been awarded a three-year EPSRC grant of c. £1.5M from October 2012.
The research will exploit the strengths of two different computational modelling approaches to enable a computer to gain “understanding” of a text. The research links computational linguistics, cognitive science and category theory, a branch of mathematics, which the team has applied to computational linguistics.
Dr. Stephen Clark, is Principal Investigator on the project at the University of Cambridge Computer Laboratory.
What is unique about this particular research?
Historically there have been two ways of representing meaning in Computational Linguistics and Natural Language Processing (NLP). The first goes back to the work of philosophers of language, such as Frege and Russell, and uses logic to represent the meanings of sentences. This is useful for a computer because logical representations are, in some sense, more precise than natural language, and have a well-founded mechanism for reasoning. The problem is that it has proven extremely difficult to represent the meanings of sentences of naturally occurring text, such as newspaper text and text from Wikipedia, in this way. The other method represents the meanings of words as vectors in a high-dimensional "meaning space", and is driven more from an empirical, data-focused perspective. Going back to the philosophical angle, we might loosely say that this approach is driven more by the Wittgensteinian philosophy of "meaning as use". The idea is quite simple: the meaning of a word like "dog" can be represented in terms of the words which tend to occur in the contexts of "dog"; words like "pet", "fluffy", "walk", "sleep" and so on. The context words can be obtained empirically by automatically analysing large amounts of text, e.g. the one billion words on Wikipedia. If we perform a similar analysis for the word "cat", we find that "dog" and "cat" tend to share context words, and hence appear close together in the information space. Words that are close together are considered to be close in meaning. The two approaches are very different, and have opposing strengths and weaknesses: the logical approach has a method for combining the meanings of words and phrases to obtain the meaning of a whole sentence, but has little to say about the meanings of individual words. The distributional, vector-based approach has a lot to say about the meanings of words, but little work has been done on how to combine them to obtain vector-based representations for phrases and sentences. In our research we are addressing the difficult question of how to combine the two approaches, for which there is surprisingly little existing work.
What does your research involve?
The 5-site grant covers a range of expertise, with mathematical work being carried out at the Universities of Oxford and York; empirical, applications-driven work at the Universities of Cambridge and Sussex; and work on the more cognitive/psychology side at the University of Edinburgh. At Cambridge, we're interested in how these sophisticated meaning representations can be used to improve performance for language processing applications; for example building better search engines or better automatic translation systems. At the heart of the research is the idea that the meanings of phrases and sentences can be compared automatically for similarity, just by observing how close the respective vectors are in the information space. Knowing whether two phrases or sentences are similar in meaning would be useful for almost all language processing applications; for example, suppose a user enters the query "cheap cars for sale" into a search engine, and on the web is a relevant page describing how "bargain automobiles can be purchased here". Humans can easily see the relevance, but a computer needs to be given lots of knowledge to know that automobiles are cars, bargain cars are cheap, and so on. The big insight which was made 20 years or so in Natural Language Processing is that much of this knowledge can be acquired automatically from text, rather than relying on laborious, manual work from linguistic experts.
Looking to the future, how do you see things developing?
In some ways the work is highly speculative, and it's refreshing that the EPSRC is willing to fund high-risk research of this kind. On the theoretical side, it is still an open question how the more traditional questions of natural language semantics can be incorporated in the vector-space framework; to give one example, how should negation (words like "not") affect the position of a vector in the information space? I expect there to be progress in this area, which will be of interest to linguists and philosophers, as well as computer scientists. On the applications side, there is a general move in the research community to incorporate more sophisticated meaning approaches into the data-driven techniques which are currently dominant (and will remain so). Our work could be considered in this vein. For example, the Google translation engine is conceptually very simple: it finds phrases in one language which tend to be seen with phrases in another language, across a large body of human-translated text. One place where these simple models fail, however, is when the corresponding phrases appear in different parts of the sentence, because word-orders differ in different languages. This is an example of where the computer requires more linguistic knowledge. So far it has been difficult to improve language technology using linguistic theory. Personally, I would like to see more Linguistics in Natural Language Processing, because I am interested in the mathematical theory of languages, but whether Linguistics, and how much of it, will ultimately prove to be useful for natural language applications is an empirical question. After all, who said that engineers need to use the whole of Physics to build bridges?